How To Build Cell Culture Models With Purpose
By Bettina Knapp, Boehringer Ingelheim
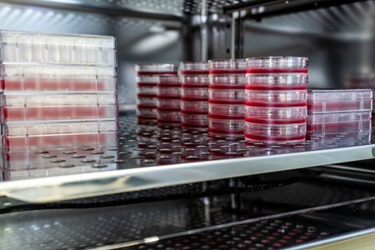
Cell culture development is a crucial aspect of the biopharmaceutical industry where the quality by design (QbD) approach is used to ensure consistently good quality of products. QbD involves a systematic approach to process understanding and control of critical process parameters and critical quality attributes.1, 2 However, in upstream processing, particularly in mammalian cell cultures, the processes and cellular behavior are often complex and not yet fully understood.
To bridge this gap, models are used to achieve desired development goals. Regardless of the model type, e.g., statistical, mechanistic, or hybrid, there are commonly three primary aims of a model:
- greater robustness of the process by gaining a better process understanding,
- faster development timelines, and
- greater sustainability with fewer experiments.
These goals may compete with each other, such as for an accelerated timeline, experiments may need to be parallelized, and learnings cannot be transferred from one experiment to another, in some cases resulting in the need for more experiments in total. However, the process must be robust and scalable. Therefore, it is important to have these goals in mind before starting with modeling, as this helps to define the model's purpose.
Define The Purpose Of The Model As The First Step
Defining the purpose of a model should always be the first crucial step in the modeling process. It helps to determine the appropriate type of model to use and which experiments to conduct.3 The purpose of a model can vary and may include process optimization, process characterization, showing process robustness, monitoring, achieving process insight, and more. Different models may show different criteria, such as the possibility of extrapolation, generalizability, explainability, reproducibility, and representativeness, depending on the model's purpose.
However, every model has limitations and makes certain assumptions. Thus, it is essential to understand these limitations and the underlying assumptions as well as to be critical in the model evaluation process. For example, if a model is very complex and difficult to interpret, it may not be used in practice, as it is not understood in detail or is not trusted and, thus, it is not useful. Therefore, it is important to correctly design experiments, interpret model results, and include appropriate risk reduction strategies.
Identifying the model’s limitations often depends on the model itself and on the underlying assumptions and the data, which are often not easy to assess. It is also dependent on the model's purpose and whether the limitations and the risks are acceptable or not. The impact of model uncertainty can vary for different model purposes. It is important to thoroughly evaluate the impact of model uncertainty on product quality and to define an approach to reduce associated risk if the uncertainty is not acceptable. Asking the following questions can be helpful in evaluating the impact of model uncertainty:
- Which decisions are going to be triggered based on the model?
- What is the impact of the decisions?
- What could go wrong?
If the model needs to be validated, the appropriate validation steps can also be different for different model types.4 Depending on the developmental phase, a higher model uncertainty might be acceptable. However, for later phases, such as in process characterization studies, this should be minimized to ensure accurate modeling and reduce associated risks for the intended commercial process.
In summary, defining the purpose of a model is a crucial step in the modeling process, as it helps to determine the appropriate type of model to use and which experiments to conduct. Understanding model limitations and the impact of model uncertainty is also important in accurately evaluating the model's effectiveness and reducing associated risks.
Good Data Should Fit The Model’s Purpose
In the process of cell culture development, it is crucial to start with data rather than experiments. Historical data or prior knowledge may already be available, and it is important to screen this data first to determine if it is sufficient for the purpose at hand. If the available data is not sufficient, it is important to investigate the reasons for this, such as inadequate analytical methods, incomplete or erroneous data, or a lack of variation in the main influencing factors.
Once the gaps in the data have been identified, experiments can be designed to fill these gaps and best address the development goal, which is the model’s purpose. However, it is important to note that not all data is good data, and a large data set is not always sufficient. To judge whether data is good, it must be analyzed systematically, looking for systematic errors, missing values, wrong data assignments, wrong data values, outliers, and other factors. Additionally, metadata, such as project, analytical method, operator, equipment, and more must be provided.
Depending on the purpose of the model, even a small number of cell culture runs may be sufficient if they are designed in a design-of-experiments manner. However, a large data set with only setpoint runs, showing no variation in input settings, will not be suitable for machine learning or statistical models.
In summary, starting with data rather than experiments is crucial in the process of cell culture development. It is important to screen available data first and investigate the reasons for any gaps before designing experiments to fill these gaps and best address the development goal. Systematic analysis of data and the provision of metadata are also important factors in determining the suitability of data for modeling purposes.
The Importance Of Model Thinking
When it comes to solving complex problems or understanding complex systems, it is not just on the modelers to create accurate models. It is important for everyone involved to understand the concept of model thinking and how it can be applied to achieve the desired outcomes.
Often, people are not clear about what a model can deliver and what it should fulfill. This can lead to misunderstandings and ineffective use of models. Therefore, it is crucial to embed models into the process and communicate effectively to ensure that everyone is on the same page. The use of models and the model interpretation should be easier than just doing more experiments. This comes along with incentivization, which is a very personal thing. It might be hard to explain why the additional effort of documenting all the metadata for the laboratory staff is so important if there is no value in the generated model for them. However, everyone is part of the process, and avoiding unnecessary experiments results in more time for doing the right experiments with all the details.
It is also important to note that complex questions or systems do not necessarily require complex solutions or calculations. Model thinking can help simplify the problem and identify the key variables that need to be considered. This helps to understand which data is needed and, even more important, to avoid performing experiments that do not address the underlying question.
To ensure that all interfaces as well as all different disciplines are included, it is important to go beyond just creating models and instead adopt a model thinking approach. This implies that all people involved in a project, i.e., laboratory staff, project managers, modelers, and so on, have transparency about the model’s purpose. This involves understanding the problem, identifying the key variables, creating a model that accurately represents the system (fit for purpose), and using the model to inform decisions. To be clear, not all people involved have to, for example, create the model, but they should all be aware of the model process, what the model can do, and what it cannot. To clearly communicate the risks and limitations of the model is as important as the model itself.
Model thinking is a crucial tool for solving complex problems and understanding complex systems. It is not just on the modelers to create accurate models, but everyone involved needs to understand the concept and how it can be applied to achieve the desired outcomes. To do so, one could think about having “modeling ambassadors” among the laboratory staff. This is just one example to build a bridge between different disciplines like the laboratory staff and the modelers. Sharing success stories about the use of models might be another step toward model thinking.
Conclusion
Modeling for cell culture or more general bioprocesses is a crucial tool in the field of biotechnology, but it comes with its own set of challenges. Pain points in cell culture modeling include the availability and formatting of data with metadata, having a clear question and purpose, and the need for interdisciplinary collaboration to embed the models in the processes.
However, the gains of effective cell culture modeling are numerous. These include optimization of processes, a holistic understanding of the system, and the definition of proven acceptable ranges and critical attributes. By using models in our processes, we can be faster, better, and more sustainable.
We should be clear about the purpose of the model and the associated risks and limitations. By doing so, we can ensure that the model is used effectively and that the desired outcomes are achieved.
To achieve these gains, it is important to consider models where they haven't been used before.
Good data is key, and it is important to be aware that all metadata is needed to ensure accurate modeling. Communication and collaboration between experts are crucial, and to avoid silos, modeling should not be treated as a separate discipline. Incentivization is also needed to encourage interdisciplinary collaboration. In the future, we will still need experiments because, without data, there cannot be modeling. However, the way we prioritize and execute experiments will change. For this, all disciplines in bioprocess development have to work together to achieve modeling goals. Then, there will be more time for the “fun” part: developing robust, efficient, and sustainable processes in an accelerated manner.
References
- Sommeregger, W.; Sissolak, B.; Kandra, K.; Von Stosch, M.; Mayer, M.; Striedner, G. Quality by control: Towards model predictive control of mammalian cell culture bioprocesses. Biotechnol. J. 2017, 12, 12.
- ICH. ICH Guideline Q8 (R2) on Pharmaceutical Development; EMA/CHMP/ICH/167068/2004; ICH, Ed.; ICH: London, UK, 2009.
- ICH PtC to Q8/Q9/Q10; 2011
- Rajamanickam, V., Babel, H., Montano-Herrera, L., Ehsani, A., et al. About model validation in bioprocessing. Process 2021, 9, 961.
About the Author:
Bettina Knapp, Ph.D., works in Boehringer Ingelheim’s biopharmaceutical area. Initially, she was responsible for drug substance and drug product stage 3 validation and led the biostatistics team in process control. Later, she became a senior associate director of CMC statistics where she was involved in authority and customer inspections in biopharmaceutical contract manufacturing. During the last five years, she has worked in biopharmaceutical development, especially focusing on upstream development and fostering the integration of models and digital twins.